Recently, Dr. He Youwei’s team from the School of Mechanical Engineering, University of South China, published a paper titled “An efficient boundary prediction method based on multi - fidelity Gaussian classification process for class - imbalance” in Engineering Applications of Artificial Intelligence. This top journal belongs to the first-tier category of the Chinese Academy of Sciences in computer science.The paper presents a boundary prediction approach using multi-fidelity Gaussian classification. It aims to cut modeling costs and boost accuracy when dealing with class imbalance. Postgraduate students Liu Lingzhi and Tan Kuan contributed to the work. Dr. He Youwei serves as the corresponding author, with the School of Mechanical Engineering, University of South China, listed as the affiliated institution.Boundary prediction is crucial in engineering design. Predicting boundaries between failure and safety limit states ranks among the field’s most popular research topics.
Physical experiments are costly and time - consuming. Thus, surrogate - model - based boundary prediction methods are commonly adopted for quick boundary estimation. Among surrogate models, the Gaussian Classification Process (GPC) is widely used. However, GPC relies solely on high - fidelity data, which are expensive to collect, driving up modeling data acquisition costs. To address this, the Multi - fidelity Gaussian Classification Process (MGPC) has been developed based on the multi - fidelity modeling paradigm.MGPC first captures the overall trend of engineering systems using abundant low - cost, low - fidelity sample points. It then refines the model with a small number of high - cost, high - fidelity ones, cutting down modeling time while maintaining accuracy.Conventionally, GPC employs Markov Chain Monte Carlo Sampling (MCMC) for training. This approach leads to heavy computational loads and slow convergence. Thus, we propose an enhanced MGPC model (MGPC - SVGD) based on Stein Variational Gradient Descent (SVGD).
In engineering boundary prediction, MGPC is vulnerable to class imbalance caused by issues like sample acquisition difficulties or uneven boundary partitioning, degrading model accuracy. To tackle this, we integrate a hybrid sampling method—combining Synthetic Minority Over - sampling Technique (SMOTE) and Edited Nearest Neighbors (ENN). This reduces the class imbalance rate of modeling data and enables the establishment of an MGPC - based boundary prediction method, as depicted in the following figure.
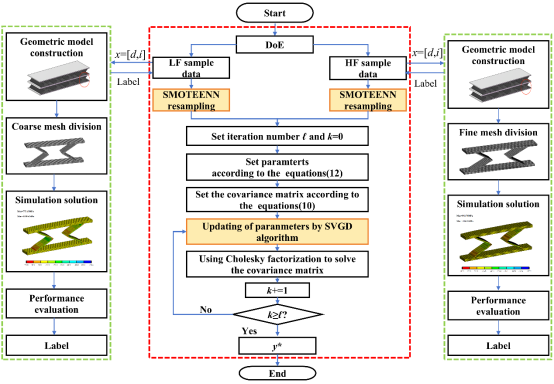
Process of Multi-fidelity Gaussian Classification Process-based Boundary Prediction Method
The proposed method is applied to predict the failure boundary of the near-zero Poisson's ratio structure and the safe operation boundary of the compressor rotor. The prediction results are shown in the following two figures. Both the modeling accuracy and speed of the proposed method are superior to those of existing models, which verifies the effectiveness and engineering practicality of the developed method. The proposed method can also provide a new approach for predicting the boundaries of the feasible region for the multi-fidelity Bayesian optimization algorithm.
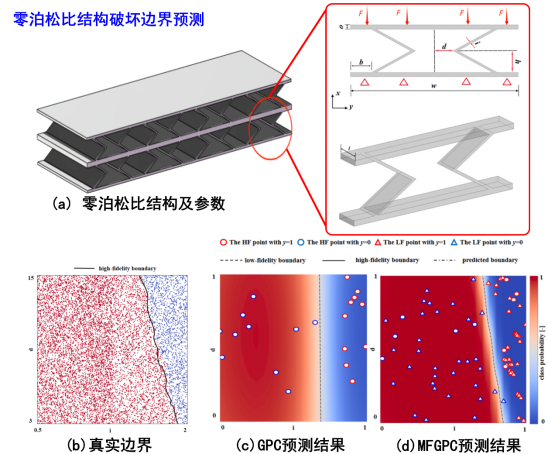
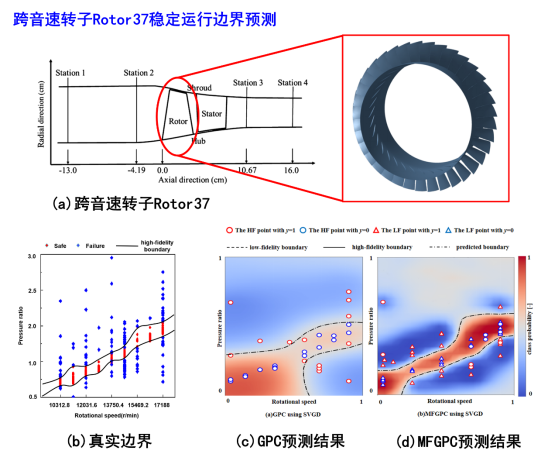
Applications of Boundary Prediction Based on the Multi-fidelity Gaussian Classification Process
This research was completed with the financial support of the Youth Project of the Natural Science Foundation of Hunan Province, the Key Project of the Scientific Research Fund of the Education Department of Hunan Province, and the Doctoral Startup Fund Project of the University of South China.
Paper link:https://doi.org/10.1016/j.engappai.2025.110549